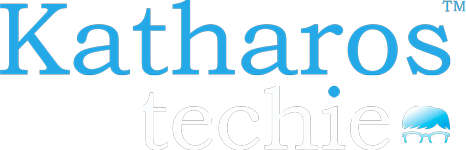
Predictive Analytics In Healthcare – 10 Use Cases and Real-World Examples
By Udit Agarwal
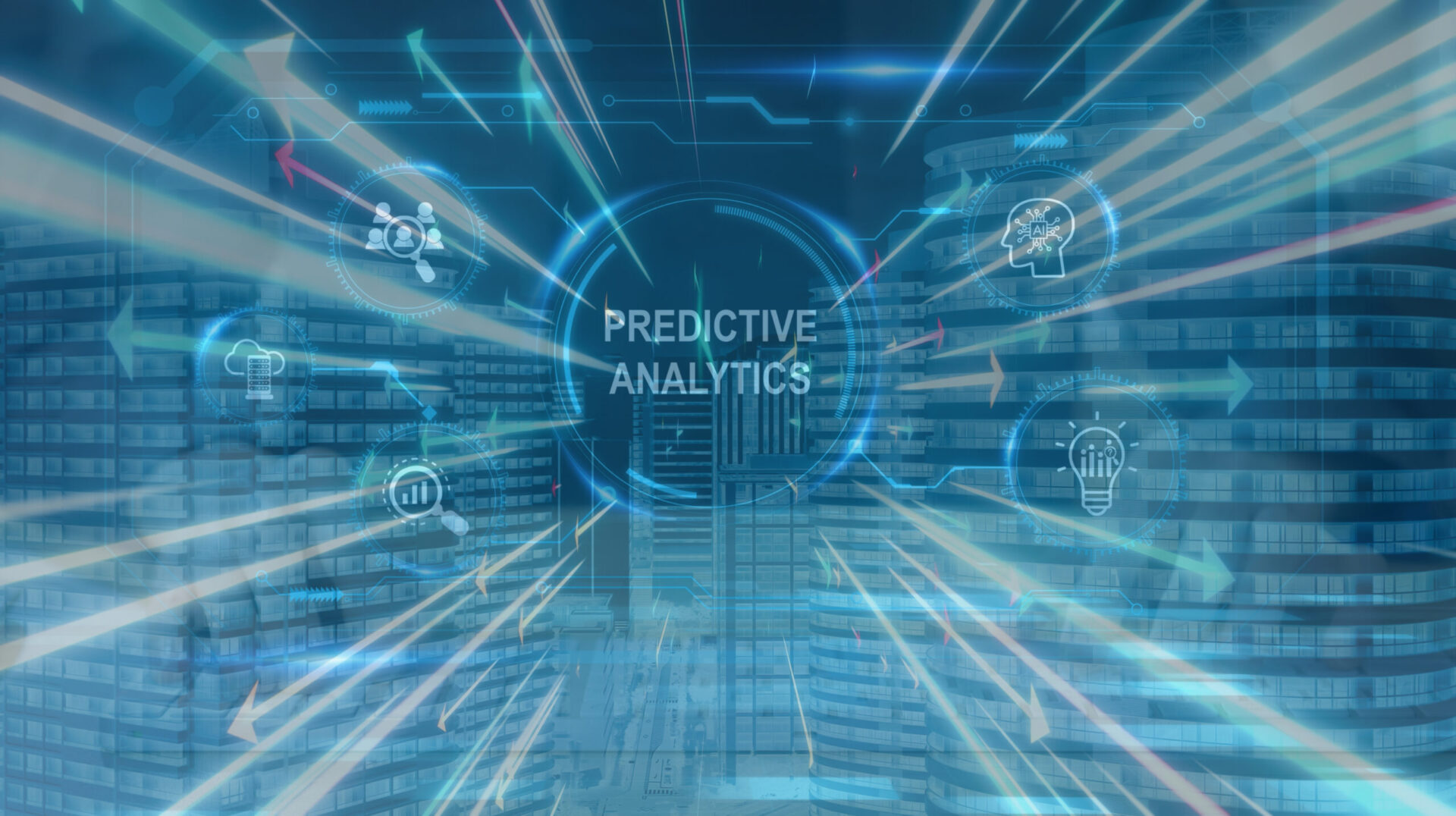
In recent years, predictive analytics has emerged as a powerful healthcare tool, revolutionizing how providers deliver care, manage resources, and improve patient outcomes. By leveraging advanced algorithms and machine learning techniques, predictive analytics enables healthcare organizations to anticipate and address medical conditions before they escalate, optimize treatment plans, and enhance operational efficiency. This article explores ten use cases of predictive analytics in healthcare, along with real-world examples illustrating their impact.
Early Disease Detection and Prevention:
Predictive analytics can help healthcare providers identify individuals at risk of developing chronic diseases such as diabetes, hypertension, and heart disease. By analyzing patient data such as demographics, medical history, and lifestyle factors, predictive models can predict the likelihood of disease onset and recommend preventive measures. For example, the American Heart Association’s Cardiovascular Health Risk Calculator uses predictive analytics to assess an individual’s risk of developing cardiovascular disease based on age, gender, blood pressure, cholesterol levels, and smoking status.
Hospital Readmission Prediction:
Reducing hospital readmissions is a crucial priority for healthcare providers aiming to improve patient outcomes and reduce healthcare costs. Predictive models can analyze patient data to identify individuals at high risk of readmission and intervene with targeted interventions to prevent unnecessary hospitalizations. For instance, the University of Pittsburgh Medical Center (UPMC) implemented a predictive analytics solution that uses electronic health record (EHR) data to identify patients at risk of readmission within 30 days of discharge, allowing care teams to implement proactive interventions such as post-discharge follow-up calls and home health visits.
Medication Adherence Monitoring:
Non-adherence to medication regimens is a common challenge in healthcare, leading to suboptimal treatment outcomes and increased healthcare costs. Predictive analytics can help healthcare providers monitor patient adherence patterns and intervene when adherence issues arise. For example, AiCure, a healthcare technology company, uses predictive analytics and artificial intelligence to monitor medication adherence in real-time by analyzing patient behavior captured through smartphone cameras. The system alerts care teams if patients fail to adhere to their medication schedule, enabling timely intervention to address adherence issues.
Patient Risk Stratification:
Patient risk stratification involves categorizing patients into risk groups based on their likelihood of experiencing adverse health events. Predictive models can analyze patient data to stratify individuals into high-, moderate–, and low-risk categories, allowing healthcare providers to allocate resources more effectively and tailor interventions to each risk group. For instance, Kaiser Permanente uses predictive analytics to stratify its patient population based on their risk of hospitalization, enabling care teams to prioritize high-risk patients for targeted interventions such as care management and care coordination.
Predictive Diagnostics:
Predictive analytics can enhance diagnostic accuracy by analyzing patient data to identify patterns and trends indicative of underlying medical conditions. For example, IBM’s Watson for Oncology uses predictive analytics to analyze patient data, medical literature, and clinical guidelines to assist oncologists in diagnosing and treating cancer. By analyzing a patient’s medical history, genomic data, and tumor characteristics, Watson for Oncology can provide personalized treatment recommendations based on the latest evidence and best practices.
Healthcare Resource Allocation:
Predictive analytics can help healthcare organizations optimize resource allocation by forecasting demand for healthcare services and identifying areas of inefficiency. For example, hospitals can use predictive models to predict patient admissions, emergency department visits, and surgery volumes, allowing them to adjust staffing levels, bed capacity, and equipment availability accordingly. Predictive analytics can help healthcare organizations identify opportunities to reduce costs and improve operational efficiency by analyzing supply chain management, inventory levels, and equipment utilization.
Chronic Disease Management:
Managing chronic diseases such as diabetes, asthma, and chronic obstructive pulmonary disease (COPD) requires ongoing monitoring and proactive intervention. Predictive can help healthcare providers identify patients at risk of disease exacerbations and intervene with targeted interventions to prevent complications and hospitalizations. For example, Propeller Health, a digital health company, uses predictive analytics to monitor asthma and COPD patients’ symptoms and medication usage, allowing care teams to intervene when patients are at risk of exacerbations.
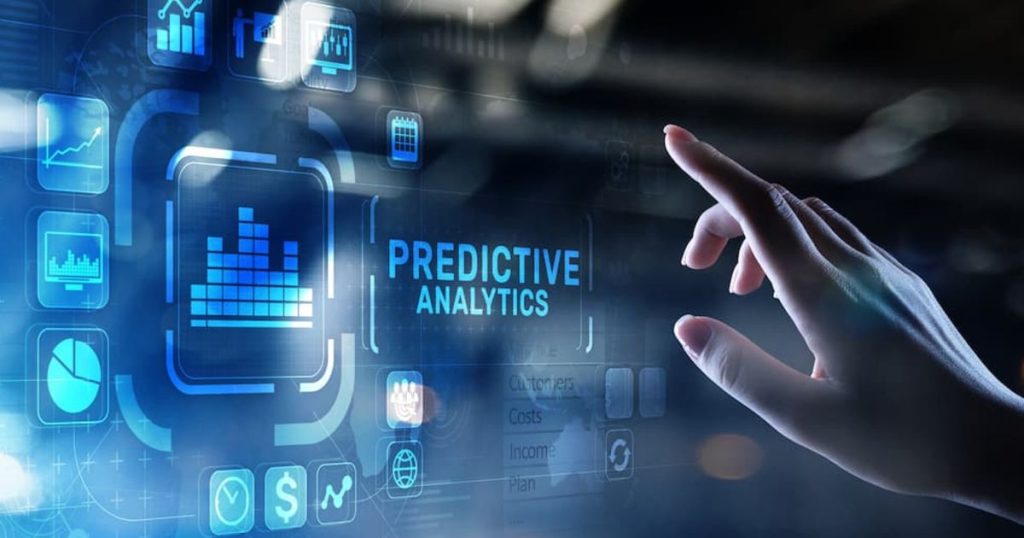
Patient Engagement and Behavior Modification:
Predictive analytics can help healthcare providers engage patients in their care and encourage behavior modification by delivering personalized interventions and incentives. For example, digital health platforms like Livongo Health use predictive analytics to analyze patient data and offer health coaching, education, and feedback to individuals with chronic conditions. By analyzing patient data such as blood glucose levels, activity levels, and medication adherence, these platforms can identify patterns and trends indicative of potential health risks and provide timely interventions to help patients manage their conditions more effectively.
Telehealth Triage and Remote Monitoring:
Telehealth and remote monitoring have become increasingly prevalent in healthcare, enabling providers to deliver care to patients remotely and monitor their health status outside of traditional healthcare settings. Predictive analytics can enhance telehealth triage and remote monitoring by analyzing patient data such as vital signs, symptoms, and medical history to identify patients at risk of deterioration or complications. For example, remote patient monitoring platforms such as Current Health use analytics to analyze patient data collected from wearable and home monitoring devices, allowing care teams to detect early warning signs of health deterioration and intervene proactively.
Precision Medicine and Personalized Treatment:
Precision medicine aims to deliver targeted therapies and interventions tailored to individual patient characteristics, including genetic makeup, biomarkers, and lifestyle factors. Predictive analytics plays a crucial role in precision medicine by analyzing patient data to identify optimal treatment strategies and predict treatment responses. For example, Foundation Medicine uses analytics to analyze tumor genomic data and identify targeted therapy options for cancer patients based on their tumor’s molecular profile. By leveraging analytics, precision medicine approaches can improve treatment outcomes, minimize adverse effects, and enhance patient satisfaction.
In conclusion, predictive analytics is promising to transform healthcare by enabling providers to anticipate and address medical conditions more effectively, optimize treatment plans, and enhance operational efficiency. By leveraging advanced algorithms and machine learning techniques, healthcare organizations can harness the power of predictive analytics to improve patient outcomes, reduce healthcare costs, and drive innovation in care delivery. As the healthcare industry continues to evolve, predictive analytics will play an increasingly vital role in shaping the future of healthcare delivery and improving patient care worldwide.