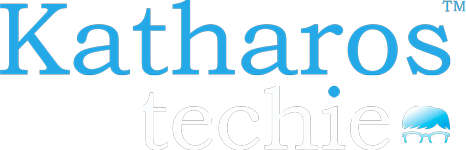
How NLP is Making Chatbots More Human-Like in Mobile Apps
By Udit Agarwal
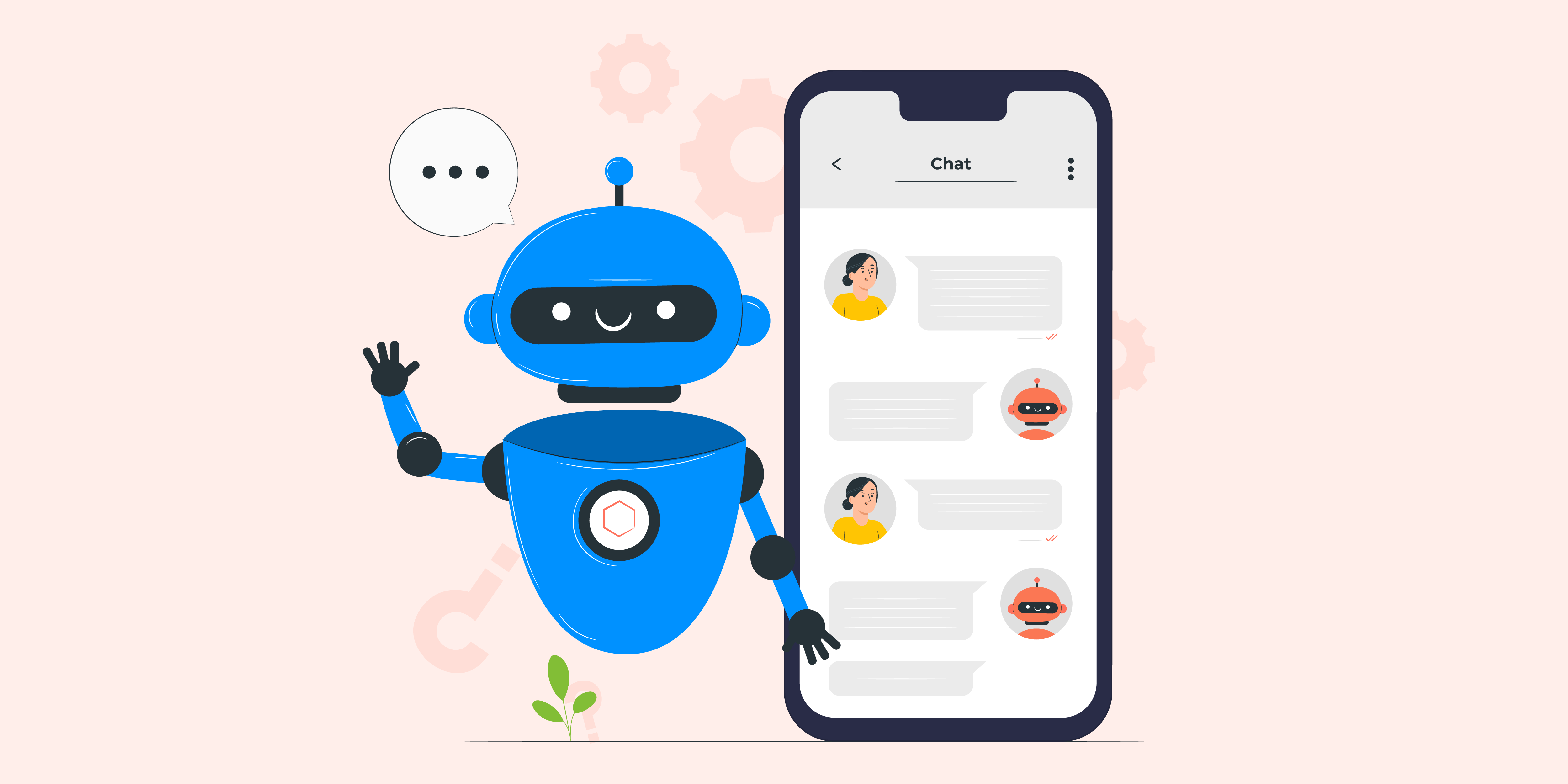
Natural Language Processing (NLP), a subfield of artificial intelligence, has revolutionized how machines understand and interact with human language. One of its most compelling applications is the development of chatbots, which have become increasingly prevalent in mobile applications. As NLP technologies advance, chatbots evolve from simple, rule-based systems to sophisticated, human-like conversational agents. This transformation enhances user experiences, streamlines customer service, and opens new avenues for interaction and engagement in mobile apps.
The Evolution of Chatbots
Chatbots have come a long way from their early iterations, often limited to answering predefined questions with scripted responses. These early chatbots operated on basic if-then logic, making them inflexible and frequently frustrating for users seeking more complex interactions. However, the integration of NLP has dramatically changed this landscape.
Modern chatbots leverage NLP to understand, interpret, and generate human language in a contextually relevant and coherent way. This shift has allowed chatbots to handle more complex queries, engage in meaningful conversations, and provide personalized responses. The evolution of NLP technologies, including advancements in machine learning, deep learning, and neural networks, has been instrumental in this transformation.
How NLP Enhances Chatbot Functionality
- Understanding Context: One of the most significant advancements in NLP is understanding context. Early chatbots struggled with context retention, often failing to maintain a coherent conversation over multiple exchanges. NLP enables chatbots to track the context of a conversation, remember previous interactions, and use that information to provide relevant responses. This capability is crucial for creating a seamless and natural conversational experience.
- Intent Recognition: NLP allows chatbots to accurately identify user intents, even when questions or commands are phrased differently. This involves parsing the language, identifying key entities, and understanding the user’s goal. For example, if a user says, “I’m looking for a nearby restaurant,” the chatbot can recognize the intent to find dining options and provide appropriate recommendations.
- Sentiment Analysis: Sentiment analysis, a component of NLP, enables chatbots to detect the emotional tone of a user’s message. Chatbots can adjust their responses by understanding whether a user is happy, frustrated, or neutral. This emotional intelligence helps provide empathetic and supportive interactions, enhancing user satisfaction.
- Language Generation: Advanced NLP models, such as OpenAI’s GPT-3 and ChatGPT, excel at generating human-like text. These models can produce coherent and contextually appropriate responses, making conversations with chatbots feel more natural and engaging. They can also handle diverse topics, providing users with informative and relevant answers across various domains.
Applications in Mobile Apps
The integration of NLP-powered chatbots in mobile apps spans a wide range of applications:
- Customer Support: Many companies deploy chatbots in their mobile apps to provide instant customer support. These chatbots can handle common queries, troubleshoot issues, and guide users through processes, reducing the need for human intervention and speeding up response times. For example, telecom companies use chatbots to assist with billing inquiries and technical support.
- E-commerce: In e-commerce apps, chatbots serve as virtual shopping assistants, helping users find products, providing recommendations, and facilitating transactions. By understanding user preferences and browsing history, these chatbots can offer personalized shopping experiences, boosting sales and customer loyalty.
- Healthcare: Healthcare apps increasingly utilize chatbots to provide medical information, schedule appointments, and offer preliminary diagnoses based on user symptoms. NLP allows these chatbots to understand medical terminology and provide accurate, relevant information, enhancing patient care and accessibility.
- Banking and Finance: Chatbots in banking apps assist with checking account balances, transferring funds, and providing financial advice. They can handle sensitive information securely and offer timely assistance, improving user convenience and trust in digital banking services.
Making Chatbots More Human-Like
The goal of making chatbots more human-like involves several strategies:
- Conversational Design: Effective conversational design is essential for creating intuitive and engaging interactions. This involves scripting dialogues that mimic natural human conversation, including casual language, humor, and empathy.
- Personalization: Personalization is critical to making interactions feel more human. By leveraging user data, chatbots can tailor their responses to individual preferences and past interactions, making users feel understood and valued.
- Continuous Learning: NLP-powered chatbots can continuously learn from new interactions, improving performance. This involves training on diverse datasets and incorporating user feedback to refine their conversational abilities.
- Voice Integration: Integrating voice recognition and synthesis technologies allows chatbots to engage in spoken conversations. This adds a layer of human-like interaction, as users can communicate with chatbots using natural speech, enhancing accessibility and user engagement.
Challenges and Future Directions
Despite significant advancements, making chatbots indistinguishable from humans remains challenging. Understanding nuanced language, handling ambiguous queries, and maintaining coherence over long conversations remain complex problems. Additionally, ethical considerations, such as ensuring transparency and preventing misuse, are critical in developing and deploying chatbots.
The future of NLP in chatbots is promising, with ongoing research focused on improving language models, enhancing contextual understanding, and developing more sophisticated sentiment analysis. As these technologies evolve, chatbots in mobile apps will become even more capable, offering more prosperous, more human-like interactions.
In conclusion, NLP transforms chatbots from simple code-based systems to sophisticated conversational agents. By enhancing understanding, context, and personalization, NLP makes chatbots more human-like, enriching user experiences across various mobile applications. The potential for even more seamless and natural interactions between humans and machines will only grow as this technology progresses.