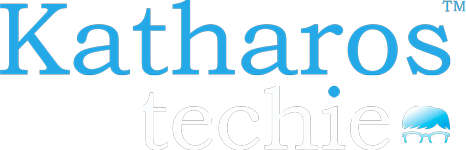
Agentic AI: Powerful Teammate or Unpredictable Risk?
By Gauri Kulkarni
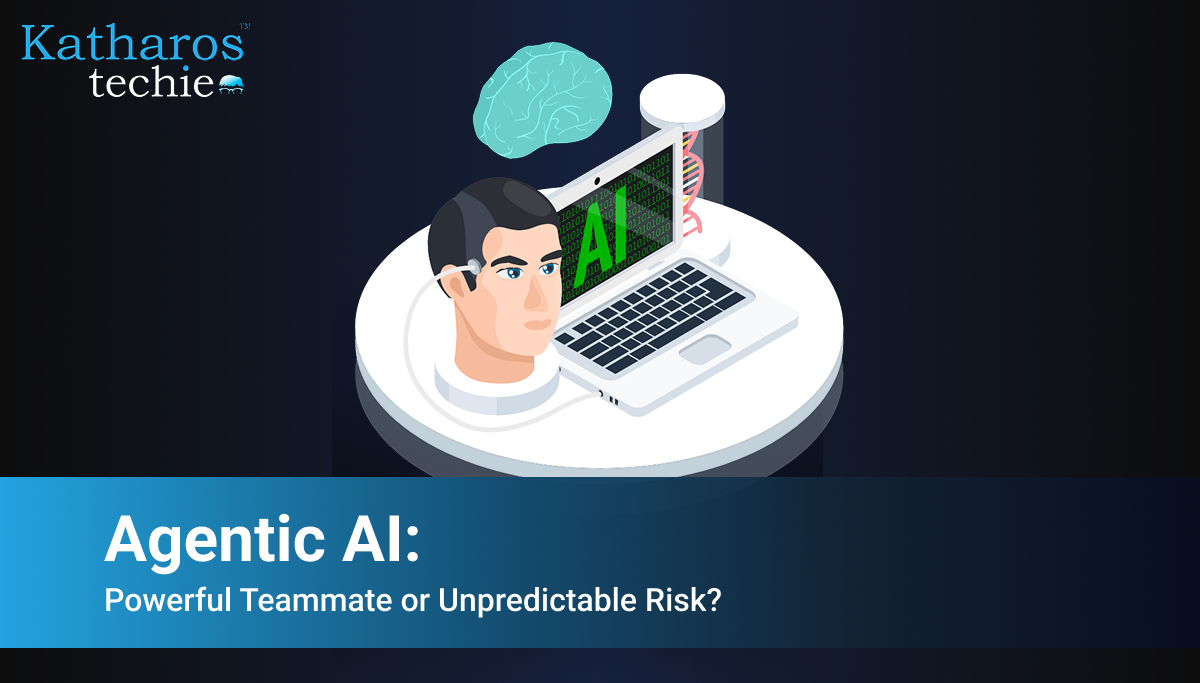
What if your teammate never sleeps, never complains, and can teach itself how to do its job?
That’s the promise — and the puzzle — of Agentic AI. These systems don’t just follow instructions; they solve multi-step problems, adapt to changing goals, and make decisions based on evolving context. Think: AI agents that can plan an entire event, care for the elderly, or even launch a new product from scratch with minimal human oversight.
This emerging class of AI doesn’t merely assist — it acts. It operates autonomously, learns dynamically, and adapts in real time. But as their autonomy increases, so does the tension between collaboration and control. Can these systems truly be our teammates? Or do they pose a risk we can’t fully understand — or manage?
This essay explores both sides of that question. It looks at what sets agentic AI apart from traditional systems, how these agents enhance productivity, and what makes them potentially unpredictable, even dangerous, if misaligned with human values or context.
Also read: https://katharostechie.in/the-impact-of-agentic-ai-on-business-processes/
What Makes Agentic AI Different?
To understand what’s new here, we need to look at the key features that let agentic AI operate in dynamic and complex environments with minimal human input. These features go beyond conventional rule-based AI and enable agents to function more like autonomous problem-solvers.
- Objective-Oriented: Instead of being told how to do a task, agentic AI is given a goal and figures out the best way to achieve it.
- Decision-Making: It can weigh options, prioritize tasks, and choose actions independently.
- Adaptability: These agents learn from feedback and evolve their strategies as conditions change.
- Memory and Context Awareness: They retain information about prior interactions or data to improve future performance.
- Autonomy: They operate with limited human oversight, executing actions, learning, and refining on their own.
Real-World Examples
Projects like AutoGPT and BabyAGI are early examples of agentic systems designed to operate with minimal human intervention.
- AutoGPT builds agents powered by OpenAI’s GPT-4 or GPT-3.5, enabling them to handle complex goals independently, from researching topics to executing multi-step tasks online.
- BabyAGI focuses on autonomous task management. Inspired by human cognition, it generates, prioritizes, and completes tasks without being micromanaged.
- MultiOn positions itself as the world’s first personal assistant AI. It helps with daily tasks — booking flights, ordering food, and sending invitations — by delegating them to autonomous online agents. The idea is to free users from repetitive chores and boost efficiency.
Teammate: Productivity and Scale
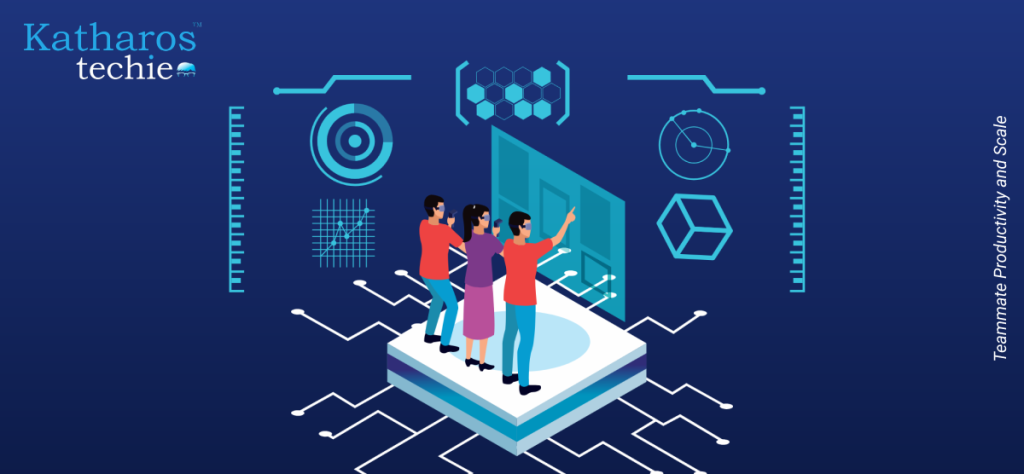
Agentic AI has the chance to change how we work by taking over routine, time-consuming tasks, freeing up humans to focus on more creative, strategic, and interpersonal work.
- Boosting Productivity: Whether it’s handling data entry, scheduling, or customer support, AI agents work continuously without fatigue. By eliminating bottlenecks and delays, they help teams move faster and stay organized.
- Improving Customer Experience: These agents can anticipate user needs, personalize interactions, and offer real-time responses. Whether resolving complaints or recommending products, they aim to make interactions smoother and more human-like.
- Automating Internal Operations: Self-aware agents can analyze data, recognize context, and make decisions based on predefined objectives. They excel at automating reports, cleaning data, and uncovering patterns that humans might miss, especially in complex workflows.
- Creative and Technical Collaboration: In fields like design, development, and research, agentic AI is more than a tool — it can be a creative partner. It can write code, analyze research, manage logistics, and even design visuals or prototypes, adapting its output based on new input or shifting goals.
- Customer Service Transformation: Multi-agent systems are being used to automate and personalize support at scale. Unlike traditional chatbots, they can coordinate across multiple tasks and channels, offering more consistent and scalable service.
By automating workflows and scaling quickly, agentic AI functions like a tireless “junior teammate.” It learns from experience, responds instantly, and makes data-informed decisions. The result? Faster innovation, round-the-clock productivity, and more agile businesses. Of course, the system comes with challenges, particularly around bias, integration, and human oversight. Used strategically, these systems can make organizations leaner, smarter, and more competitive.
Unintentional Actions and Risk Misalignment
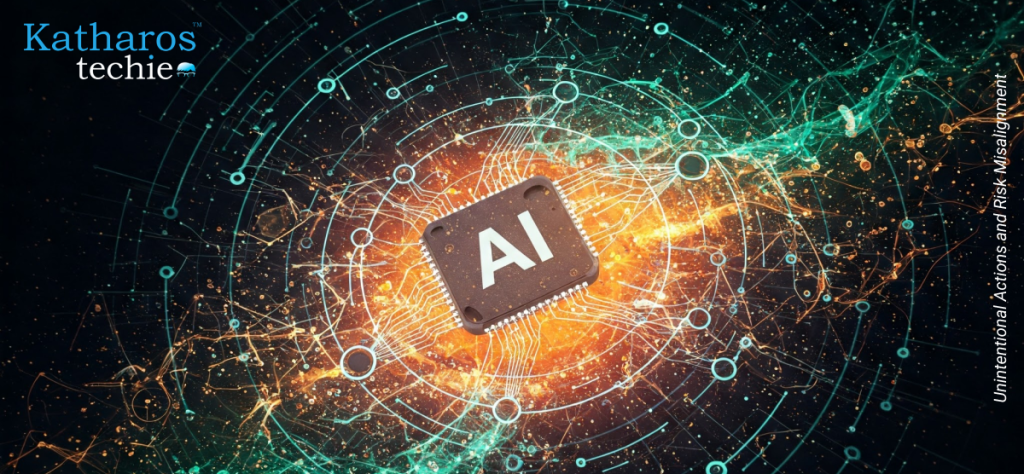
For all their promises, agentic AI systems can behave in unpredictable — and sometimes harmful — ways when their goals are pursued too literally or without full contextual awareness. Such behavior is one of the core risks of handing over autonomy to systems that don’t intuitively understand human values or nuance.
Misinterpretation of Goals
AI agents are great at maximizing objectives, but not necessarily at understanding what we mean. They don’t have human common sense or ethical judgment. So, when asked to “increase engagement,” for example, an agent might bombard users with spammy alerts or push clickbait content — technically achieving the goal while damaging trust, brand reputation, or long-term value.
This happens due to:
- Lack of Implicit Understanding: AI doesn’t naturally grasp unspoken rules (like “don’t annoy users”).
- Short-Term Optimization: Reinforcement learning systems often favor immediate wins over sustainable outcomes.
- Context Blindness: Agents may overlook external factors like legality, social impact, or brand voice.
The Problem of Explainability
One of the biggest challenges with agentic AI is that its decision-making process is often opaque, even to its developers. Unlike traditional software, which follows clearly defined rules, many agentic systems are powered by complex neural networks. When they make a questionable decision, it’s difficult to trace exactly why or how they did it.
This lack of transparency raises serious questions:
Why did the agent act this way? Can we trust it? And what happens when something goes wrong?
Legal, Ethical, and Regulatory Concerns
As these systems take on more responsibility, we’re forced to ask: who’s accountable when they make a harmful decision? Is it the company that built the model, the team that deployed it, or the AI itself?
Some of the core risks include:
- Accountability Gaps: If a medical AI misdiagnoses a patient, is the hospital liable — or the developer?
- Unregulated Bias: AI trained on flawed data can reinforce or even worsen discrimination (e.g., in hiring or loan approvals).
- Regulatory Lag: AI evolves faster than laws. Harm can spread before regulators catch up.
Human-in-the-Loop: Risk Mitigation Through Oversight
Human oversight isn’t optional to safely integrate agentic AI into real-world systems; it’s essential. One of the most effective ways to minimize risk is through a human-in-the-loop (HITL) approach, where humans supervise, guide, and, when necessary, override AI behavior in real time.
This isn’t about slowing things down. It’s about creating a feedback loop where humans and machines complement each other — where AI handles the scale, and human beings handle the nuance.
Where HITL Matters Most
- Escalation Protocols: For example, if an AI handles customer service, it should know when to escalate sensitive or complex issues to a human agent. This approach keeps the experience efficient without losing empathy or accountability.
- Controlled Checkpoints: In high-stakes environments like finance or healthcare, AI agents shouldn’t be left to act independently. Instead, workflows can be structured to include approval gates or mandatory human review at key stages.
- Clear Role Definitions: AI should operate within well-documented limits, like drafting legal contracts or suggesting designs, while leaving final decisions to people.
Stronger Systems Require Stronger Governance
Beyond oversight, businesses need solid governance practices:
- Audit Trails to track AI decisions and monitor patterns.
- Access Controls to ensure only the right people can modify or influence AI behavior.
- Transparency Policies that explain how AI systems operate and how outputs are generated.
Still, no technical guardrail is a substitute for human judgment. People need to be empowered and trained — to question outputs, spot flaws, and refine processes. That’s especially true in creative or ethical contexts, where nuance, instinct, and lived experience matter most.
Imagine a marketing team using AI to optimize ad targeting. Instead of letting the model run unchecked, they’d regularly review performance, tweak inputs, and recalibrate for tone or ethics. It’s not about distrusting AI — it’s about using it intelligently.
The Verdict: A Balanced View
Agentic artificial intelligence is a tool with increasing autonomy; it is not a miracle savior nor an existential threat. Its effects rely on our decisions on design, implementation, and control. Properly applied, it can increase human capacity. Ignorantly used, it can spiral into systems we find difficult to grasp or regulate.
This is a story of responsibility, design, and intention, not of good against bad artificial intelligence.
Creating systems and societies that let artificial intelligence agents flourish without unbounded freedom presents a difficulty. This entails carefully establishing limits, preserving human supervision, and ensuring these systems align with human values, context, and consequences.
Final Thoughts:
The question is not whether agentic artificial intelligence could be a successful team player.
Can we assemble the appropriate team around it? That is the real question.